Brian Moore, February 7, 2021
Get the Best Solution for
Your Business Today!
Table of Contents
Introduction
With the rollout of Planning Analytics Workspace Local 2.0.57 (and 2.0.58 in Cloud), IBM released the long-anticipated Predictive Forecasting capabilities. This allows users to generate forecast data based on historical actuals within the cube. Sophisticated statistical algorithms calculate behind the scenes to provide accurate time series projections which blend trends and seasonality. This out-of-the-box functionality is a huge value add for organizations looking to begin down the path of objective predicted forecasts instead of previous error prone methods overly reliant on human intuition and biases. Predictive Forecasting separates IBM Planning Analytics as a market leader over competing vendors as this feature has traditionally required additional software. Along with dashboarding capabilities, the new Applications & Plans, Excel reporting features, and industry leading performance, IBM continues to develop Planning Analytics into a one-stop-shop for best-in-class financial performance management.
How Does It Work?
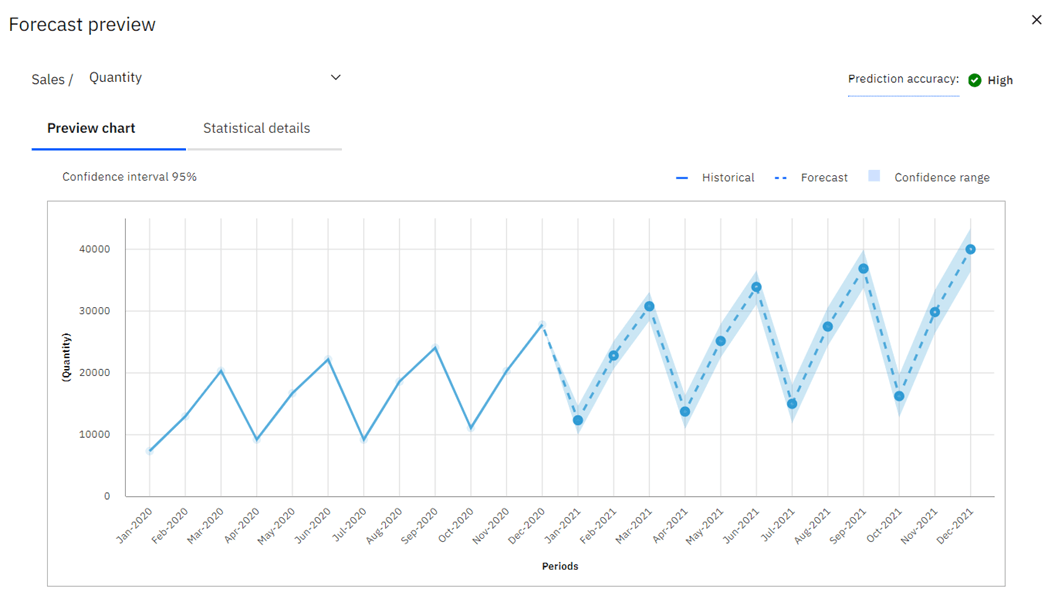
Users can perform self-service predictions in PAW with ease. Simply drag a reporting cube view onto the canvas and orient so that time period data is located on columns. The cube can contain one continuous time dimension or two separate stacked time dimensions (Year and Month); both cube architectures will work. One particular item that has caused some confusion is aggregations/rollups within time dimensions. Many organizations may have Total Year rollups or YTD rollups of months, and these rollups confuse the algorithms. This can be resolved by building a separate hierarchy in the Periods dimension containing only input level periods and using this new hierarchy in the cube view for the prediction.
Traditionally the rows dimension should contain items in which a time series and seasonality-based prediction would provide value. Product sales by unit, net income by location, and revenue by customer are a few predictive scenarios common to most organizations.
Once the cube view is properly oriented, highlight a row for the item in which you are predicting and press the icon (pictured below) located on the far right of the top toolbar.
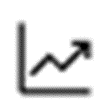
The Forecast wizard will pop out on the right side of the screen and users will be able to enter settings for the forecast. Settings include selecting the range of future time periods to be forecasted, whether seasonality should be auto detected, and setting the confidence interval (90/95/99%). Once settings have been selected, users can choose to Preview or Forecast. Preview prepares a visualization and statistical results whereas Forecast commits the data to the cube. Note that several rows can be highlighted at the same time for a Forecast, and as of PAW 2.0.60 multiple rows can be previewed as well. Be aware of the data volume when running forecasts on large consolidations as this may tie up system resources and have long calculation times.
Is It Right for Me?
Absolutely! Predictive forecasting will benefit all Planning Analytics customers regardless of industry. Retail, Manufacturing, Energy, Banking, Insurance, etc.; every customer will have a use case where time series forecasting will provide some degree of insight into future performance. One potential pitfall regarding the accuracy of the results is the lack of historical data available. It is preferable that 2 years of historical data are available for the algorithms to provide the most accuracy, and an additional rule of thumb is to have twice as many historical periods as the number of periods you are seeking to predict. Having less than two years of data might not be enough for proper detection of seasonal patterns that only occur once per year. An additional problem area for accurate predictions are data sets that do not contain trends or seasonality. There are some business use cases where the data is truly random; often due to low data volume, which can lead to subpar predictions.
Other than these two scenarios, Predictive Forecasting will likely provide excellent statistical based projections of future performance. No longer will the organization rely on a linear percentage increase of prior year data, or haphazard executive adjustments to back into a randomly selected total. The algorithm based objective approach continues to become the new standard in financial and operational planning.
Closing
In summary, the recent release of Predictive Forecasting in PAW will provide great benefits to your financial planning processes. Not only will customers see improved accuracy, but also a much-improved cycle time. Having accurate baselines provided by an algorithm-based process saves planners from the busy work of having to put thought into each data point in the forecast. Instead, just a cursory review for outliers should be enough to quickly provide an accurate submission of the plan.
Next Steps
The team at PMsquare has resources available to upgrade PA Local clients to the latest version of PAW, as well as deliver training on these new features. In addition, we will continue to offer more info on the latest releases of Planning Analytics Workspace in the form of YouTube Livestreams, Planning Analytics tutorials, and future blog posts so be sure to check out our YouTube channel at https://www.youtube.com/pmsquare and our website www.pmsquare.com.
Please reach out to review the Budgeting and Planning goals of your organization and what is needed to introduce Predictive Forecasting today!
We hope you found this article informative. Be sure to subscribe to our newsletter for data and analytics news, updates, and insights delivered directly to your inbox.